Recent history has shown the current energy system is vulnerable to disruptions in the fuel supply chains, leading to high and unpredictable natural gas and electricity prices. The path towards a more resilient energy system is the green energy transition, which can only be expected to accelerate from now on. The accelerated green transition is a new paradigm that the district energy sector needs to adapt to with an increasingly forward-looking response.
By Jan Eric Thorsen, Director, Global Application, Emanuele Zilio, Solution Engineer, Danfoss,
Tomaz Benedik, Solution Manager, Danfoss, and Oddgeir Gudmundsson, Director, Projects, Danfoss
Published in Hot Cool, edition no. 2/2023 | ISSN 0904 9681 |
Many district heating systems have taken important steps in the last decades, such as consolidating multiple local networks into one large system and operating multiple and varied heat sources instead of the traditional single-source approach. They are also transitioning from high-temperature to low-temperature operation.
Those steps enable efficient operation of, e.g., heat pumps for taking advantage of locally available waste energy and low-temperature renewable thermal sources and moving away from fossil fuels. While the systems are inevitably becoming vastly more complex, they are also opening an enormous opportunity for holistically optimizing the whole system from one end to the other.
The question is how to deal with the increased complexity this transformation brings. In Danfoss, we see the solution in the extensive use of digitalization and AI throughout the entire district energy supply chain; planning of a new system, extension of an existing system or modernization and maintenance of existing systems; strategic location of new heat plants and optimizing the heat generation and network operation in multi-source systems based on reliable demand forecasts; continuous operating parameter optimization of building installations, utilization of the building thermal mass and reliable demand forecasting of each building.
With an end-to-end focus, the full potential for leveraging the flexibility of the district heating infrastructure can be unlocked. This will enable the efficient use of a wide variety of heat sources, such as data centers, supermarkets, Power-to-X, Carbon Capture, industry, wastewater treatment, and renewable sources, such as biomass, wind, solar thermal, or geothermal energy.
This union of flexibility, efficiency, and adoption of intelligent digital tools significantly increases the resilience of the district heating infrastructure. The impact reaches far beyond the district heating systems, making the whole energy system smarter, more efficient, and more reliable.
For this reason, we are continuously digitalizing our hardware portfolio to create smart components upgraded with advanced artificial intelligence (AI) based software solutions that maximize the value of information for helping utilities and building owners to make better and fact-based decisions.
Danfoss solutions
The outcome of our digitalization journey is a portfolio of software and services under the umbrella of Leanheat for the control and optimization of district energy systems, from the producer to the consumer. The Leanheat software suite includes four different solutions.
- Leanheat Production: An advanced software for load forecasting, planning, and optimizing district energy production and distribution. The cornerstone of Leanheat Production is a six-day AI-based demand forecast. The software calculates the cost-optimal production mix from available heat sources based on the estimates and energy spot prices.
- Leanheat Network: A thermo-hydraulic modeling tool developed specifically to support district energy system planning, design, and operation. With the help of the Leanheat Network digital twin in the planning and design process, the cost of establishing new and modifying existing district energy systems can be minimized. Once in operation, the digital twin will support the district heating utilities by optimizing the operation, leading to lower operational expenses.
- Leanheat Monitor: A dedicated software for efficient remote monitoring, optimizing, and managing substations and heating installations. Leanheat Monitor further simplifies collecting and visualizing data that the district heating utility can use to optimize its operation. By using the software, district heating utilities can remotely detect faults or wrong settings and perform tasks that before required on-site intervention – thereby resulting in time and cost savings.
- Leanheat Building: A software solution for optimizing the operation of heating installations of buildings with a centralized heating system. It utilizes the latest AI and machine learning developments to generate accurate thermodynamic models of the buildings it controls. It combines indoor climate monitoring and weather forecast to achieve energy savings and decrease the volatility of indoor temperature associated with traditional heating control strategies, improving living conditions for occupants. Furthermore, the control algorithm can optimize consumption and shift load while maintaining indoor comfort.
Case example: Flexumers project in Copenhagen with HOFOR and Copenhagen City Properties & Procurement
An example of a Danfoss software application is to be found in Copenhagen. Here HOFOR (district heating utility), Copenhagen City Properties & Procurement (Municipality’s building department), and Danfoss are currently testing the potential of minimizing peak heating demand to increase CO2 neutral base-load heat production usage in Copenhagen by utilizing Leanheat Building AI-based heating control.
The first part of the demonstration took place during the heating season 2021/2022 and included 17 municipal buildings (mainly daycare centers). The buildings were already equipped with Danfoss communicative heating controllers, and they were connected to the Leanheat AI control via the Danfoss ECL portal.
The main goal of the demonstration was to reduce the peak in heat demand that occurs in the mornings (6-10 am) by making the heat consumption more flexible. Thus, the project has been named district heating Flexumers since the buildings that previously were only seen as energy consumers have become an active part of the district heating system. Each building acts as a virtual heat plant by increasing its consumption when heat production is cheap and ecological and decreasing during times of high demand by providing flexibility on the consumption side.
Leanheat’s AI learns how the building’s thermal mass reacts to ambient conditions and evaluates the flexibility potential based on the forecasted weather and set comfort requirements. The district heating utility can then use the estimated flexibility to minimize the load during peak-load periods. For example, during the morning peak, the control allows the discharging of the heat previously stored in the buildings by reducing the supply temperature for space heating by up to 30%.
After this, it is recharged as soon as possible before the following day. The variation of supply temperature during this period still ensures that the thermal comfort in the building stays within the recommended limits.
As presented in Figure 1, the initial results of the demonstrations for the cluster of 17 buildings show that the average morning peak decreased by 14%, compared with the average peak consumption before the implementation of the smart control.
The connected buildings also reduced their heating energy consumption, predictively factoring in upcoming changes in weather, such as solar radiation and wind. Based on the demonstration results, the overall economic and environmental benefits of Flexumers in Copenhagen will be quantified by mid-2023.
The peak demand data measured at the buildings in Figure 2 show that the maximum peak power has decreased from 27.5 kW/building to 21.5 kW/building (-22%). The calculation compares the highest peak during load shifting to the highest measured peak in the previous heating season at the same outdoor conditions.
HOFOR sees district heating Flexumers as an important measure to minimize fossil-based peak load production and incentivize renewable-based heat-producing units. The same principle of peak power optimization can be utilized in demand-response solutions for district heating concerning charging and discharging the thermal energy in the building mass.
Leanheat can offer flexibility from an aggregated building stock, making it possible for the district heating company to produce heat and use it in the buildings when it is most beneficial economically or ecologically.
The DH company will thus reduce the use of fossil-based heat sources and prioritize renewable-based ones. For example, with the advanced knowledge of the building thermal mass, Leanheat Building can enable price signals to adjust the building heat supply to take advantage of low-cost periods, for example, when there is a large share of fluctuating renewable energy in the system.
Case example: optimization of domestic hot water storage tank control
Having data available from communicative components or controllers gives a high degree of freedom for testing and validating new functionalities. Besides the heating supply to the building, the domestic hot water (DHW) system is also relevant for improving performance based on digitalization and AI.
As part of the HEAT 4.0 project (Digitally supported Smart District Heating, IFD ref. no.: 8090-00046B), the operation of DHW storage tanks was analyzed to develop a control method for reducing the district heating return temperature and the peak power. One of the test site installations is shown in figure 3.
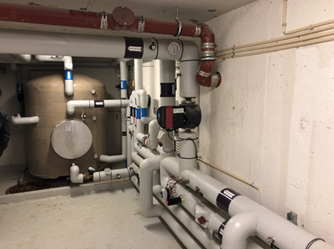
Figure 3. DHW storage tank with an internal heating coil (background) and heating mixing shunt (foreground)
Often DHW tank applications suffer from high district heating return temperatures and high-power peaks. This typically relates to poor controller settings, wrong control valve sizing, poor heat transfer via the tank coil, poor DHW stratification, and a high share of DHW circulation loss compared to DHW tapping.
To improve the performance of DHW tank applications, a new functionality was developed for the Danfoss ECL controllers. The new functionality is an intelligent district heating return temperature limiting function. It works so that when a pre-set return temperature is exceeded, the charging flow to the tank coil is reduced, and thus the district heating return temperature will be kept below the pre-set value.
In the same way, there is the option for a power limiting function, limiting the district heating charging power when a pre-set value is exceeded. While the functionality sounds straightforward, it has certain complexities in determining an appropriate setting. A too high setpoint results in no or limited engagement of the function, and a too low setpoint compromises the DHW temperature.
Further, the optimal setpoint varies over the year due to seasonal variations in the cold-water temperature and the district heating supply temperature. The ratio of the DHW consumption and DHW circulation will also influence the optimal settings. Examples of seasonal variations are given in the figures below:
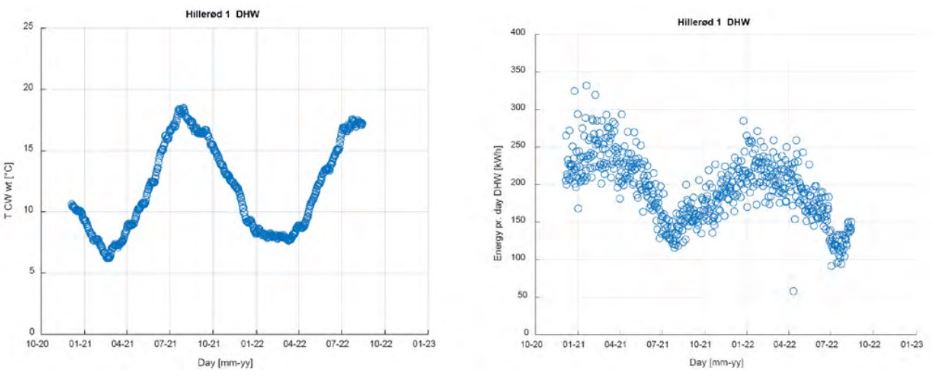
Figure 4. Seasonal variation of cold-water temperature (left) and energy used for preparing DHW (right)
As Figure 4 shows, the cold water varies by 12°C during the year, and the daily DHW demand varies by ~+/-35% of the annual average DHW demand. Based on these variations, it’s understandable that the setting is not straightforward.
An adaptive method was developed to address this challenge. The adaptive return temperature principle continuously adjusts the controller settings and adapts to the actual boundary conditions. An example where the adaptive control is compared to the reference control can be seen in Figure 5.
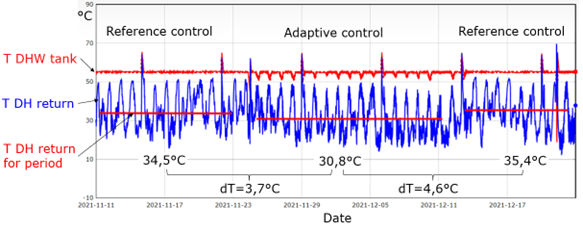
Figure 5. Comparing district heating return-temperature during adaptive control and reference control periods
In the shown example, a reduction of the district heating return temperature of 3.7°C and 4.6°C was realized, compared to pre- and post-reference periods, respectively. The temperature spikes are related to the disinfection of the DHW system, which has a similar impact on the reference and adaptive control periods. Because of the control principle, the DHW temperature is slightly reduced in short periods during the adaptive control period, engaging the limiter function in an optimal way.
In addition to the adaptive district heating return temperature limiting function, a power limiting function was developed. This control principle requires an energy meter to measure the power supplied to the DHW tank, which is becoming more applied due to general energy awareness and heat cost allocation.
An example is given in Figure 6, where a reduced district heating return temperature of 6.2°C and 6.7°C was realized. Further, the power (magenta line) generally shows fewer and lower peaks during the adaptive control periods.
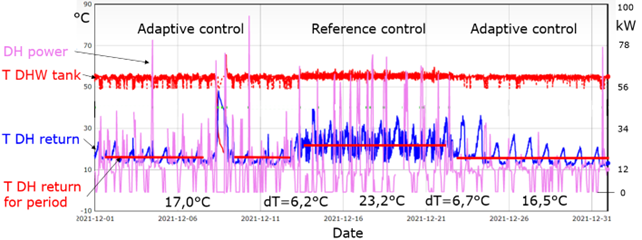
Figure 6 Comparing district heating return temperature and power peaks during adaptive and reference control periods
Based on tests in six buildings, the realized flow-weighted return temperature reduction is, on average, around 2°C for the adaptive return temperature limiter and around 4°C for the adaptive power limiter annually. This is a significant decrease in the district heating return temperature for the service of DHW. Regarding peak power reduction, a 25-30% reduction is realized based on 30 min average values.
Conclusion
The energy transition is high on the agenda, and it’s great to see how district energy is a key enabler and a fundamental part of the future smart and sector-coupled energy system. Digitalization and AI-based tools are a precondition for district energy systems’ efficient, cost-optimal, and resilient operation.
The Copenhagen Flexumers case showed an average capacity reduction of 14% during peak load hours, leading to better utilization of green resources, avoidance of fossil-based peak load boilers, and better utilization of the existing distribution infrastructure.
The example of optimizing the DHW tank charging resulted in a reduced return temperature of 2-4°C and 25-30% peak power reduction, leading to distribution heat loss savings, better utilization of the energy source and is often a precondition for reducing the supply temperature or increase of the distribution capacity.
The presented cases are two of many underlining that we are continuously developing new and improving existing products and offerings at Danfoss. We believe digitalization and AI-based end-to-end control solutions are the keys to unlocking the grid’s full potential and realizing District Energy 4.0.
For further information please contact: Jan Eric Thorsen, jet@Danfoss.com